|
|
Click the serial number on the left to view the details of the item. |
# |
Author | Title | Accn# | Year | Item Type | Claims |
11 |
Conti, Claudio |
Quantum Machine Learning |
I12944 |
2024 |
eBook |
|
12 |
Sutton, Andrew T.C |
Domain Generalization with Machine Learning in the NOvA Experiment |
I12894 |
2023 |
eBook |
|
13 |
Bufano, Filomena |
Machine Learning for Astrophysics |
I12856 |
2023 |
eBook |
|
14 |
Lista, Luca |
Statistical Methods for Data Analysis |
I12774 |
2023 |
eBook |
|
15 |
Guo, Huaqun |
IRC-SET 2022 |
I12721 |
2023 |
eBook |
|
16 |
Yao, Kan |
Nanophotonics and Machine Learning |
I12686 |
2023 |
eBook |
|
17 |
Joshi, Nirav |
Machine Learning for Advanced Functional Materials |
I12655 |
2023 |
eBook |
|
18 |
Lin, Kang-Ping |
Future Trends and Challenges of Molecular Imaging and AI Innovation |
I12506 |
2022 |
Book |
|
19 |
Martin Perez, Cristina |
Search for the Higgs Boson Produced in Association with Top Quarks with the CMS Detector at the LHC |
I12497 |
2022 |
Book |
|
20 |
Abaimov, Stanislav |
Machine Learning for Cyber Agents |
I12486 |
2022 |
Book |
|
|
11.
|
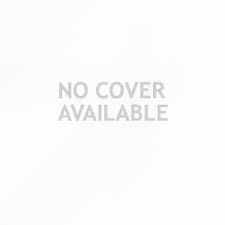 |
Title | Quantum Machine Learning : Thinking and Exploration in Neural Network Models for Quantum Science and Quantum Computing |
Author(s) | Conti, Claudio |
Publication | Cham, 1. Imprint: Springer
2. Springer International Publishing, 2024. |
Description | XXIII, 378 p. 109 illus., 66 illus. in color : online resource |
Abstract Note | This book presents a new way of thinking about quantum mechanics and machine learning by merging the two. Quantum mechanics and machine learning may seem theoretically disparate, but their link becomes clear through the density matrix operator which can be readily approximated by neural network models, permitting a formulation of quantum physics in which physical observables can be computed via neural networks. As well as demonstrating the natural affinity of quantum physics and machine learning, this viewpoint opens rich possibilities in terms of computation, efficient hardware, and scalability. One can also obtain trainable models to optimize applications and fine-tune theories, such as approximation of the ground state in many body systems, and boosting quantum circuits??? performance. The book begins with the introduction of programming tools and basic concepts of machine learning, with necessary background material from quantum mechanics and quantum information also provided. This enables the basic building blocks, neural network models for vacuum states, to be introduced. The highlights that follow include: non-classical state representations, with squeezers and beam splitters used to implement the primary layers for quantum computing; boson sampling with neural network models; an overview of available quantum computing platforms, their models, and their programming; and neural network models as a variational ansatz for many-body Hamiltonian ground states with applications to Ising machines and solitons. The book emphasizes coding, with many open source examples in Python and TensorFlow, while MATLAB and Mathematica routines clarify and validate proofs. This book is essential reading for graduate students and researchers who want to develop both the requisite physics and coding knowledge to understand the rich interplay of quantum mechanics and machine learning |
ISBN,Price | 9783031442261 |
Keyword(s) | 1. Computational Physics and Simulations
2. COMPUTER SIMULATION
3. EBOOK
4. EBOOK - SPRINGER
5. MACHINE LEARNING
6. Mathematical Models of Cognitive Processes and Neural Networks
7. MATHEMATICAL PHYSICS
8. Neural networks (Computer science)??
9. QUANTUM COMPUTERS
10. Quantum computing
11. QUANTUM INFORMATION
12. QUANTUM PHYSICS
|
Item Type | eBook |
Multi-Media Links
Please Click here for eBook
Circulation Data
Accession# | |
Call# | Status | Issued To | Return Due On | Physical Location |
I12944 |
|
|
On Shelf |
|
|
|
|
12.
|
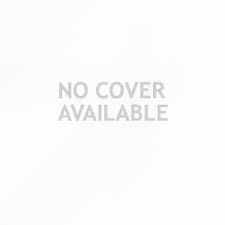 |
Title | Domain Generalization with Machine Learning in the NOvA Experiment |
Author(s) | Sutton, Andrew T.C |
Publication | Cham, 1. Imprint: Springer
2. Springer Nature Switzerland, 2023. |
Description | XI, 170 p. 73 illus., 63 illus. in color : online resource |
Abstract Note | This thesis presents significant advances in the use of neural networks to study the properties of neutrinos. Machine learning tools like neural networks (NN) can be used to identify the particle types or determine their energies in detectors such as those used in the NOvA neutrino experiment, which studies changes in a beam of neutrinos as it propagates approximately 800 km through the earth. NOvA relies heavily on simulations of the physics processes and the detector response; these simulations work well, but do not match the real experiment perfectly. Thus, neural networks trained on simulated datasets must include systematic uncertainties that account for possible imperfections in the simulation. This thesis presents the first application in HEP of adversarial domain generalization to a regression neural network. Applying domain generalization to problems with large systematic variations will reduce the impact of uncertainties while avoiding the risk of falselyconstraining the phase space. Reducing the impact of systematic uncertainties makes NOvA analysis more robust, and improves the significance of experimental results |
ISBN,Price | 9783031435836 |
Keyword(s) | 1. Accelerator Physics
2. Computational Physics and Simulations
3. COMPUTER SIMULATION
4. EBOOK - SPRINGER
5. MACHINE LEARNING
6. MATHEMATICAL PHYSICS
7. MEASUREMENT
8. Measurement Science and Instrumentation
9. MEASURING INSTRUMENTS
10. PARTICLE ACCELERATORS
11. PARTICLE PHYSICS
12. PARTICLES (NUCLEAR PHYSICS)
|
Item Type | eBook |
Multi-Media Links
media link description
Circulation Data
Accession# | |
Call# | Status | Issued To | Return Due On | Physical Location |
I12894 |
|
|
On Shelf |
|
|
|
|
13.
|
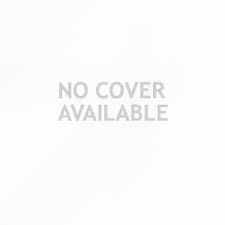 |
Title | Machine Learning for Astrophysics : Proceedings of the ML4Astro International Conference 30 May - 1 Jun 2022 |
Author(s) | Bufano, Filomena;Riggi, Simone;Sciacca, Eva;Schilliro, Francesco |
Publication | Cham, 1. Imprint: Springer
2. Springer International Publishing, 2023. |
Description | XIV, 211 p. 52 illus., 47 illus. in color : online resource |
Abstract Note | This book reviews the state of the art in the exploitation of machine learning techniques for the astrophysics community and gives the reader a complete overview of the field. The contributed chapters allow the reader to easily digest the material through balanced theoretical and numerical methods and tools with applications in different fields of theoretical and observational astronomy. The book helps the reader to really understand and quantify both the opportunities and limitations of using machine learning in several fields of astrophysics |
ISBN,Price | 9783031341670 |
Keyword(s) | 1. ARTIFICIAL INTELLIGENCE
2. ASTRONOMY
3. Astronomy, Observations and Techniques
4. ASTROPHYSICS
5. EBOOK - SPRINGER
6. MACHINE LEARNING
|
Item Type | eBook |
Multi-Media Links
media link description
Circulation Data
Accession# | |
Call# | Status | Issued To | Return Due On | Physical Location |
I12856 |
|
|
On Shelf |
|
|
|
|
14.
|
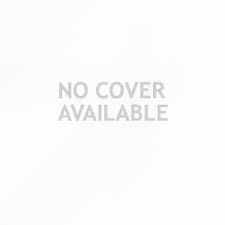 |
Title | Statistical Methods for Data Analysis : With Applications in Particle Physics |
Author(s) | Lista, Luca |
Publication | Cham, 1. Imprint: Springer
2. Springer International Publishing, 2023. |
Description | XXX, 334 p. 1 illus : online resource |
Abstract Note | This third edition expands on the original material. Large portions of the text have been reviewed and clarified. More emphasis is devoted to machine learning including more modern concepts and examples. This book provides the reader with the main concepts and tools needed to perform statistical analyses of experimental data, in particular in the field of high-energy physics (HEP). It starts with an introduction to probability theory and basic statistics, mainly intended as a refresher from readers??? advanced undergraduate studies, but also to help them clearly distinguish between the Frequentist and Bayesian approaches and interpretations in subsequent applications. Following, the author discusses Monte Carlo methods with emphasis on techniques like Markov Chain Monte Carlo, and the combination of measurements, introducing the best linear unbiased estimator. More advanced concepts and applications are gradually presented, including unfolding and regularization procedures, culminating in the chapter devoted to discoveries and upper limits. The reader learns through many applications in HEP where the hypothesis testing plays a major role and calculations of look-elsewhere effect are also presented. Many worked-out examples help newcomers to the field and graduate students alike understand the pitfalls involved in applying theoretical concepts to actual data |
ISBN,Price | 9783031199349 |
Keyword(s) | 1. Data Analysis and Big Data
2. EBOOK - SPRINGER
3. MACHINE LEARNING
4. PARTICLE PHYSICS
5. PARTICLES (NUCLEAR PHYSICS)
6. Quantitative research
7. STATISTICAL PHYSICS
|
Item Type | eBook |
Multi-Media Links
media link description
Circulation Data
Accession# | |
Call# | Status | Issued To | Return Due On | Physical Location |
I12774 |
|
|
On Shelf |
|
|
|
|
15.
|
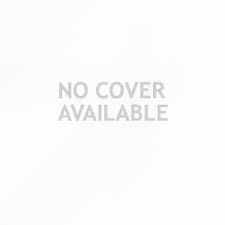 |
Title | IRC-SET 2022 : Proceedings of the 8th IRC Conference on Science, Engineering and Technology, August 2022, Singapore |
Author(s) | Guo, Huaqun;McLoughlin, Ian;Chekole, Eyasu Getahun;Lakshmanan, Umayal;Meng, Weizhi;Wang, Peng Cheng;Lu, Jiqiang |
Publication | Singapore, 1. Imprint: Springer
2. Springer Nature Singapore, 2023. |
Description | XIV, 607 p. 460 illus., 372 illus. in color : online resource |
Abstract Note | This book highlights contemporary state of research in multi-disciplinary areas in Physics, Biomedical Sciences, Chemical Engineering, Mechanical Engineering, Computer Science/Engineering, Life Sciences, and Healthcare. The accepted submissions to the 8th IRC Conference on Science, Engineering and Technology (IRC-SET 2022) that were presented on 6th August 2022, are published in this conference proceedings. The papers presented here were shortlisted after extensive rounds of rigorous reviews by a panel of esteemed individuals who are pioneers and experts in their respective domains |
ISBN,Price | 9789811972225 |
Keyword(s) | 1. Applied and Technical Physics
2. Biomaterials
3. Biomedical Devices and Instrumentation
4. Biomedical engineering
5. Biomedical Engineering and Bioengineering
6. EBOOK - SPRINGER
7. MACHINE LEARNING
8. Nanoengineering
9. NANOTECHNOLOGY
10. PHYSICS
|
Item Type | eBook |
Multi-Media Links
media link description
Circulation Data
Accession# | |
Call# | Status | Issued To | Return Due On | Physical Location |
I12721 |
|
|
On Shelf |
|
|
|
|
16.
|
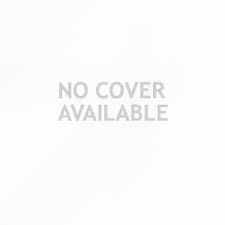 |
Title | Nanophotonics and Machine Learning : Concepts, Fundamentals, and Applications |
Author(s) | Yao, Kan;Zheng, Yuebing |
Publication | Cham, 1. Imprint: Springer
2. Springer International Publishing, 2023. |
Description | XII, 178 p. 95 illus., 92 illus. in color : online resource |
Abstract Note | This book, the first of its kind, bridges the gap between the increasingly interlinked fields of nanophotonics and artificial intelligence (AI). While artificial intelligence techniques, machine learning in particular, have revolutionized many different areas of scientific research, nanophotonics holds a special position as it simultaneously benefits from AI-assisted device design whilst providing novel computing platforms for AI. This book is aimed at both researchers in nanophotonics who want to utilize AI techniques and researchers in the computing community in search of new photonics-based hardware. The book guides the reader through the general concepts and specific topics of relevance from both nanophotonics and AI, including optical antennas, metamaterials, metasurfaces, and other photonic devices on the one hand, and different machine learning paradigms and deep learning algorithms on the other. It goes on to comprehensively survey inverse techniques for device design, AI-enabled applications in nanophotonics, and nanophotonic platforms for AI. This book will be essential reading for graduate students, academic researchers, and industry professionals from either side of this fast-developing, interdisciplinary field |
ISBN,Price | 9783031204739 |
Keyword(s) | 1. EBOOK - SPRINGER
2. MACHINE LEARNING
3. Metamaterials
4. Microwaves, RF Engineering and Optical Communications
5. Nanophotonics
6. Nanophotonics and Plasmonics
7. Plasmonics
8. TELECOMMUNICATION
|
Item Type | eBook |
Multi-Media Links
media link description
Circulation Data
Accession# | |
Call# | Status | Issued To | Return Due On | Physical Location |
I12686 |
|
|
On Shelf |
|
|
|
|
17.
|
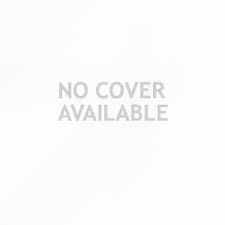 |
Title | Machine Learning for Advanced Functional Materials |
Author(s) | Joshi, Nirav;Kushvaha, Vinod;Madhushri, Priyanka |
Publication | Singapore, 1. Imprint: Springer
2. Springer Nature Singapore, 2023. |
Description | VIII, 303 p. 102 illus., 94 illus. in color : online resource |
Abstract Note | This book presents recent advancements of machine learning methods and their applications in material science and nanotechnologies. It provides an introduction to the field and for those who wish to explore machine learning in modeling as well as conduct data analyses of material characteristics. The book discusses ways to enhance the material???s electrical and mechanical properties based on available regression methods for supervised learning and optimization of material attributes. In summary, the growing interest among academics and professionals in the field of machine learning methods in functional nanomaterials such as sensors, solar cells, and photocatalysis is the driving force for behind this book. This is a comprehensive scientific reference book on machine learning for advanced functional materials and provides an in-depth examination of recent achievements in material science by focusing on topical issues using machine learning methods |
ISBN,Price | 9789819903931 |
Keyword(s) | 1. DETECTORS
2. EBOOK - SPRINGER
3. MACHINE LEARNING
4. MATERIALS
5. OPTICAL ENGINEERING
6. OPTICS
7. Optics and Photonics
8. Photonic Devices
9. PHOTONICS
10. Photonics and Optical Engineering
11. Sensors and biosensors
12. Tumor markers
13. Tumour Biomarkers
|
Item Type | eBook |
Multi-Media Links
media link description
Circulation Data
Accession# | |
Call# | Status | Issued To | Return Due On | Physical Location |
I12655 |
|
|
On Shelf |
|
|
|
|
18.
|
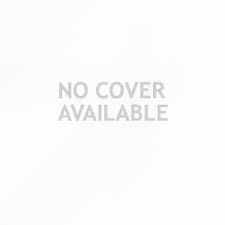 |
Title | Future Trends and Challenges of Molecular Imaging and AI Innovation : Proceedings of FASMI 2020 |
Author(s) | Lin, Kang-Ping;Liu, Ren-Shyan;Yang, Bang-Hung |
Publication | Cham, 1. Imprint: Springer
2. Springer International Publishing, 2022. |
Description | XI, 90 p. 42 illus., 36 illus. in color : online resource |
Abstract Note | This volumes presents the proceedings of the FASMI 2020 conference, held at Taipei Veterans General Hospital on November 20-22, 2020. It presents contributions on all aspects of molecular imaging, discovered by leading academic scientists and researchers. It also provides a premier interdisciplinary treatment of recent innovations, trend, and concerns as well as practical challenges and solutions in Molecular Imaging and put an emphasis on Artificial Intelligence applied to Imaging Data. FASMI is the annual meeting of the Federation of Asian Societies for Molecular Imaging |
ISBN,Price | 9783030927868 |
Keyword(s) | 1. Biology???Research
2. Biomedical engineering
3. Biomedical Engineering and Bioengineering
4. Biomedical Research
5. BIOPHYSICS
6. EBOOK
7. EBOOK - SPRINGER
8. MACHINE LEARNING
9. MEASUREMENT
10. Measurement Science and Instrumentation
11. MEASURING INSTRUMENTS
12. Medicine???Research
|
Item Type | Book |
Multi-Media Links
Please Click here for eBook
Circulation Data
Accession# | |
Call# | Status | Issued To | Return Due On | Physical Location |
I12506 |
|
|
On Shelf |
|
|
|
|
19.
|
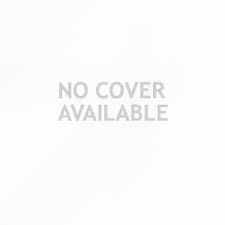 |
Title | Search for the Higgs Boson Produced in Association with Top Quarks with the CMS Detector at the LHC |
Author(s) | Martin Perez, Cristina |
Publication | Cham, 1. Imprint: Springer
2. Springer International Publishing, 2022. |
Description | XIII, 283 p. 170 illus., 158 illus. in color : online resource |
Abstract Note | In this book, the interaction between the Higgs boson and the top quark is studied with the CMS detector at the LHC via the search for the associate production of the Higgs boson with one (tH) or two (ttH) top quarks. These processes are very rare and thus a high particle selection efficiency by the trigger system is essential. The selection of hadronically decaying tau leptons, expected from the Higgs boson decays, is tackled in the first part, where the trigger is optimized for Run 2 and Run 3 and a novel machine-learning based trigger for the High-Luminosity LHC is developed. The second part presents the analysis of tH and ttH where the Higgs boson decays into tau leptons, W or Z bosons with Run 2 data. The presence of multiple particles in the final state leads to the use of multivariant discriminants based on machine learning and the Matrix Element Method. The sophisticated methods used and the unprecedented amount of data result in the most precise cross section measurements to date |
ISBN,Price | 9783030902063 |
Keyword(s) | 1. Data Analysis and Big Data
2. EBOOK
3. EBOOK - SPRINGER
4. Elementary particles (Physics)
5. Elementary Particles, Quantum Field Theory
6. MACHINE LEARNING
7. Quantitative research
8. QUANTUM FIELD THEORY
|
Item Type | Book |
Multi-Media Links
Please Click here for eBook
Circulation Data
Accession# | |
Call# | Status | Issued To | Return Due On | Physical Location |
I12497 |
|
|
On Shelf |
|
|
|
|
20.
| 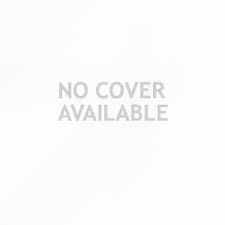 |
Title | Machine Learning for Cyber Agents : Attack and Defence |
Author(s) | Abaimov, Stanislav;Martellini, Maurizio |
Publication | Cham, 1. Imprint: Springer
2. Springer International Publishing, 2022. |
Description | XV, 227 p. 27 illus., 23 illus. in color : online resource |
Abstract Note | The cyber world has been both enhanced and endangered by AI. On the one hand, the performance of many existing security services has been improved, and new tools created. On the other, it entails new cyber threats both through evolved attacking capacities and through its own imperfections and vulnerabilities. Moreover, quantum computers are further pushing the boundaries of what is possible, by making machine learning cyber agents faster and smarter. With the abundance of often-confusing information and lack of trust in the diverse applications of AI-based technologies, it is essential to have a book that can explain, from a cyber security standpoint, why and at what stage the emerging, powerful technology of machine learning can and should be mistrusted, and how to benefit from it while avoiding potentially disastrous consequences. In addition, this book sheds light on another highly sensitive area ??? the application of machine learning for offensive purposes, an aspect that is widely misunderstood, under-represented in the academic literature and requires immediate expert attention |
ISBN,Price | 9783030915858 |
Keyword(s) | 1. Computer networks???Security measures
2. Data and Information Security
3. Data protection
4. EBOOK
5. EBOOK - SPRINGER
6. MACHINE LEARNING
7. Mobile and Network Security
8. Security Science and Technology
9. Security systems
|
Item Type | Book |
Multi-Media Links
Please Click here for eBook
Circulation Data
Accession# | |
Call# | Status | Issued To | Return Due On | Physical Location |
I12486 |
|
|
On Shelf |
|
|
|
| |