|
|
Click the serial number on the left to view the details of the item. |
# |
Author | Title | Accn# | Year | Item Type | Claims |
21 |
Guo, Huaqun |
IRC-SET 2021 |
I12441 |
2022 |
Book |
|
22 |
Madenci, Erdogan |
Advances in Peridynamics |
I12400 |
2022 |
Book |
|
23 |
Moriwaki, Kana |
Large-Scale Structure of the Universe |
I12304 |
2022 |
Book |
|
24 |
Andrejevic, Nina |
Machine Learning-Augmented Spectroscopies for Intelligent Materials Design |
I12242 |
2022 |
Book |
|
25 |
Banerjee, Santo |
Nonlinear Dynamics and Applications |
I12233 |
2022 |
Book |
|
26 |
Jiang, Richard |
Big Data Privacy and Security in Smart Cities |
I12193 |
2022 |
Book |
|
27 |
Bhatawdekar, Ramesh M |
Environmental Issues of Blasting |
I11955 |
2021 |
eBook |
|
28 |
Dickinson, Jennet Elizabeth |
ATLAS Measurements of the Higgs Boson Coupling to the Top Quark in the Higgs to Diphoton Decay Channel |
I11910 |
2021 |
eBook |
|
29 |
Tosciri, Cecilia |
Higgs Boson Decays into a Pair of Bottom Quarks |
I11867 |
2021 |
eBook |
|
30 |
Schuld, Maria |
Machine Learning with Quantum Computers |
I11858 |
2021 |
eBook |
|
|
21.
|
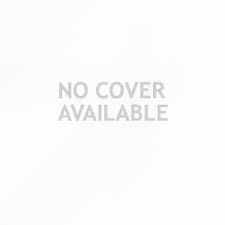 |
Title | IRC-SET 2021 : Proceedings of the 7th IRC Conference on Science, Engineering and Technology, August 2021, Singapore |
Author(s) | Guo, Huaqun;Ren, Hongliang;Wang, Victor;Chekole, Eyasu Getahun;Lakshmanan, Umayal |
Publication | Singapore, 1. Imprint: Springer
2. Springer Nature Singapore, 2022. |
Description | XVII, 693 p. 392 illus., 325 illus. in color : online resource |
Abstract Note | This book highlights contemporary state of research in multidisciplinary areas in computer science, computer engineering, chemical engineering, mechanical engineering, physics, biomedical sciences, life sciences, medicine, and health care. The accepted submissions to the 7th IRC Conference on Science, Engineering and Technology (IRC-SET 2021) that were presented on August 7, 2021, are published in this conference proceedings. The papers presented here were shortlisted after extensive rounds of rigorous reviews by a panel of esteemed individuals who are pioneers and experts in their respective domains |
ISBN,Price | 9789811698699 |
Keyword(s) | 1. Applied and Technical Physics
2. Biomaterials
3. Biomedical Devices and Instrumentation
4. Biomedical engineering
5. Biomedical Engineering and Bioengineering
6. EBOOK
7. EBOOK - SPRINGER
8. MACHINE LEARNING
9. Nanoengineering
10. NANOTECHNOLOGY
11. PHYSICS
|
Item Type | Book |
Multi-Media Links
Please Click here for eBook
Circulation Data
Accession# | |
Call# | Status | Issued To | Return Due On | Physical Location |
I12441 |
|
|
On Shelf |
|
|
|
|
22.
|
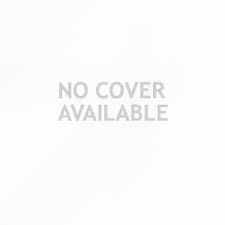 |
Title | Advances in Peridynamics |
Author(s) | Madenci, Erdogan;Roy, Pranesh;Behera, Deepak |
Publication | Cham, 1. Imprint: Springer
2. Springer International Publishing, 2022. |
Description | XVI, 421 p. 240 illus., 234 illus. in color : online resource |
Abstract Note | This book presents recent improvements in peridynamic modeling of structures. It provides sufficient theory and numerical implementation helpful to both new and existing researchers in the field. The main focus of the book is on the non-ordinary state-based (NOSB) peridynamics (PD) and its applications for performing finite deformation. It presents the framework for modeling high stretch polymers, viscoelastic materials, thermoelasticity, plasticity, and creep. It provides a systematic derivation for dimensionally reduced structures such as axisymmetric structures and beams. Also, it presents a novel approach to impose boundary conditions without suffering from displacement kinks near the boundary. Furthermore, it presents refinements to bond-based PD model by including rotation kinematics for modeling isotropic and composite materials. Moreover, it presents a PD ??? FEM coupling framework in ANSYS based on principle for virtual work. Lastly, it presents an application of neural networks in the peridynamic (PINN) framework. Sample codes are provided for readers to develop hands-on experience on peridynamic modeling. Describes new developments in peridynamics and their applications in the presence of material and geometric nonlinearity; Describes an approach to seamlessly couple PD with FE; Introduces the use of the neural network in the PD framework to solve engineering problems; Provides theory and numerical examples for researchers and students to self-study and apply in their research (Codes are provided as supplementary material); Provides theoretical development and numerical examples suitable for graduate courses |
ISBN,Price | 9783030978587 |
Keyword(s) | 1. Applied Dynamical Systems
2. DYNAMICS
3. EBOOK
4. EBOOK - SPRINGER
5. ENGINEERING MATHEMATICS
6. ENGINEERING MECHANICS
7. MACHINE LEARNING
8. MATHEMATICAL PHYSICS
9. Mechanics, Applied
10. NONLINEAR THEORIES
|
Item Type | Book |
Multi-Media Links
Please Click here for eBook
Circulation Data
Accession# | |
Call# | Status | Issued To | Return Due On | Physical Location |
I12400 |
|
|
On Shelf |
|
|
|
|
23.
|
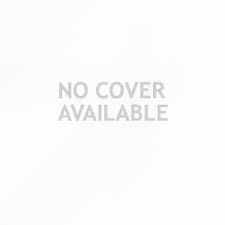 |
Title | Large-Scale Structure of the Universe : Cosmological Simulations and Machine Learning |
Author(s) | Moriwaki, Kana |
Publication | Singapore, 1. Imprint: Springer
2. Springer Nature Singapore, 2022. |
Description | XII, 120 p. 46 illus., 44 illus. in color : online resource |
Abstract Note | Line intensity mapping (LIM) is an observational technique that probes the large-scale structure of the Universe by collecting light from a wide field of the sky. This book demonstrates a novel analysis method for LIM using machine learning (ML) technologies. The author develops a conditional generative adversarial network that separates designated emission signals from sources at different epochs. It thus provides, for the first time, an efficient way to extract signals from LIM data with foreground noise. The method is complementary to conventional statistical methods such as cross-correlation analysis. When applied to three-dimensional LIM data with wavelength information, high reproducibility is achieved under realistic conditions. The book further investigates how the trained machine extracts the signals, and discusses the limitation of the ML methods. Lastly an application of the LIM data to a study of cosmic reionization is presented. This book benefits students and researchers who are interested in using machine learning to multi-dimensional data not only in astronomy but also in general applications |
ISBN,Price | 9789811958809 |
Keyword(s) | 1. Astronomy, Observations and Techniques
2. Astronomy???Observations
3. ASTROPHYSICS
4. COSMOLOGY
5. EBOOK
6. EBOOK - SPRINGER
7. MACHINE LEARNING
|
Item Type | Book |
Multi-Media Links
Please Click here for eBook
Circulation Data
Accession# | |
Call# | Status | Issued To | Return Due On | Physical Location |
I12304 |
|
|
On Shelf |
|
|
|
|
24.
|
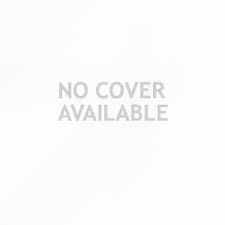 |
Title | Machine Learning-Augmented Spectroscopies for Intelligent Materials Design |
Author(s) | Andrejevic, Nina |
Publication | Cham, 1. Imprint: Springer
2. Springer International Publishing, 2022. |
Description | XII, 97 p. 29 illus., 28 illus. in color : online resource |
Abstract Note | The thesis contains several pioneering results at the intersection of state-of-the-art materials characterization techniques and machine learning. The use of machine learning empowers the information extraction capability of neutron and photon spectroscopies. In particular, new knowledge and new physics insights to aid spectroscopic analysis may hold great promise for next-generation quantum technology. As a prominent example, the so-called proximity effect at topological material interfaces promises to enable spintronics without energy dissipation and quantum computing with fault tolerance, yet the characteristic spectral features to identify the proximity effect have long been elusive. The work presented within permits a fine resolution of its spectroscopic features and a determination of the proximity effect which could aid further experiments with improved interpretability. A few novel machine learning architectures are proposed in this thesis work which leverage the case when the data is scarce and utilize the internal symmetry of the system to improve the training quality. The work sheds light on future pathways to apply machine learning to augment experiments |
ISBN,Price | 9783031148088 |
Keyword(s) | 1. EBOOK
2. EBOOK - SPRINGER
3. ELECTRONIC DEVICES
4. MACHINE LEARNING
5. SOLID STATE PHYSICS
6. SPECTROSCOPY
7. SPECTRUM ANALYSIS
8. Surfaces (Technology)
9. Surfaces, Interfaces and Thin Film
10. THIN FILMS
|
Item Type | Book |
Multi-Media Links
Please Click here for eBook
Circulation Data
Accession# | |
Call# | Status | Issued To | Return Due On | Physical Location |
I12242 |
|
|
On Shelf |
|
|
|
|
25.
|
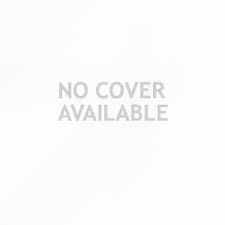 |
Title | Nonlinear Dynamics and Applications : Proceedings of the ICNDA 2022 |
Author(s) | Banerjee, Santo;Saha, Asit |
Publication | Cham, 1. Imprint: Springer
2. Springer International Publishing, 2022. |
Description | XXXI, 1474 p. 608 illus., 551 illus. in color : online resource |
Abstract Note | This book covers recent trends and applications of nonlinear dynamics in various branches of society, science, and engineering. The selected peer-reviewed contributions were presented at the International Conference on Nonlinear Dynamics and Applications (ICNDA 2022) at Sikkim Manipal Institute of Technology (SMIT) and cover a broad swath of topics ranging from chaos theory and fractals to quantum systems and the dynamics of the COVID-19 pandemic. Organized by the SMIT Department of Mathematics, this international conference offers an interdisciplinary stage for scientists, researchers, and inventors to present and discuss the latest innovations and trends in all possible areas of nonlinear dynamics |
ISBN,Price | 9783030997922 |
Keyword(s) | 1. BIOINFORMATICS
2. COMPLEX SYSTEMS
3. Computational and Systems Biology
4. DYNAMICAL SYSTEMS
5. EBOOK
6. EBOOK - SPRINGER
7. MACHINE LEARNING
8. PLASMA WAVES
9. Stochastic Networks
10. STOCHASTIC PROCESSES
11. SYSTEM THEORY
12. Waves, instabilities and nonlinear plasma dynamics
|
Item Type | Book |
Multi-Media Links
Please Click here for eBook
Circulation Data
Accession# | |
Call# | Status | Issued To | Return Due On | Physical Location |
I12233 |
|
|
On Shelf |
|
|
|
|
26.
|
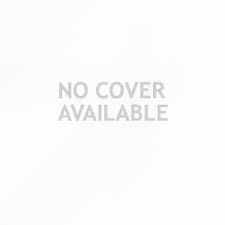 |
Title | Big Data Privacy and Security in Smart Cities |
Author(s) | Jiang, Richard;Bouridane, Ahmed;Li, Chang-Tsun;Crookes, Danny;Boussakta, Said;Hao, Feng;A. Edirisinghe, Eran |
Publication | Cham, 1. Imprint: Springer
2. Springer International Publishing, 2022. |
Description | VI, 248 p. 74 illus., 65 illus. in color : online resource |
Abstract Note | This book highlights recent advances in smart cities technologies, with a focus on new technologies such as biometrics, blockchains, data encryption, data mining, machine learning, deep learning, cloud security, and mobile security. During the past five years, digital cities have been emerging as a technology reality that will come to dominate the usual life of people, in either developed or developing countries. Particularly, with big data issues from smart cities, privacy and security have been a widely concerned matter due to its relevance and sensitivity extensively present in cybersecurity, healthcare, medical service, e-commercial, e-governance, mobile banking, e-finance, digital twins, and so on. These new topics rises up with the era of smart cities and mostly associate with public sectors, which are vital to the modern life of people. This volume summarizes the recent advances in addressing the challenges on big data privacy and security in smart cities and points out the future research direction around this new challenging topic |
ISBN,Price | 9783031044243 |
Keyword(s) | 1. Big data
2. Biometric identification
3. Biometrics
4. Cooperating objects (Computer systems)
5. Cyber-Physical Systems
6. Data protection???Law and legislation
7. EBOOK
8. EBOOK - SPRINGER
9. MACHINE LEARNING
10. Privacy
|
Item Type | Book |
Multi-Media Links
Please Click here for eBook
Circulation Data
Accession# | |
Call# | Status | Issued To | Return Due On | Physical Location |
I12193 |
|
|
On Shelf |
|
|
|
|
27.
|
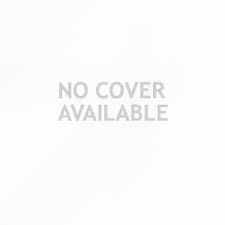 |
Title | Environmental Issues of Blasting : Applications of Artificial Intelligence Techniques |
Author(s) | Bhatawdekar, Ramesh M;Armaghani, Danial Jahed;Azizi, Aydin |
Publication | Singapore, Springer Nature Singapore, 2021. |
Description | IX, 77 p. 9 illus., 8 illus. in color : online resource |
Abstract Note | This book gives a rigorous and up-to-date study of the various AI and machine learning algorithms for resolving environmental challenges associated with blasting. Blasting is a critical activity in any mining or civil engineering project for breaking down hard rock masses. A small amount of explosive energy is only used during blasting to fracture rock in order to achieve the appropriate fragmentation, throw, and development of muck pile. The surplus energy is transformed into unfavourable environmental effects such as back-break, flyrock, air overpressure, and ground vibration. The advancement of artificial intelligence and machine learning techniques has increased the accuracy of predicting these environmental impacts of blasting. This book discusses the effective application of these strategies in forecasting, mitigating, and regulating the aforementioned blasting environmental hazards |
ISBN,Price | 9789811682377 |
Keyword(s) | 1. Computational Intelligence
2. EBOOK
3. EBOOK - SPRINGER
4. Engineering geology
5. ENVIRONMENTAL MANAGEMENT
6. Geoengineering
7. GEOPHYSICS
8. Geotechnical engineering
9. Geotechnical Engineering and Applied Earth Sciences
10. MACHINE LEARNING
|
Item Type | eBook |
Multi-Media Links
Please Click here for eBook
Circulation Data
Accession# | |
Call# | Status | Issued To | Return Due On | Physical Location |
I11955 |
|
|
On Shelf |
|
|
|
|
28.
|
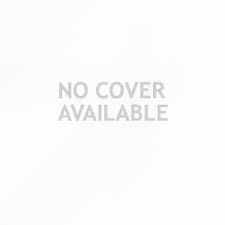 |
Title | ATLAS Measurements of the Higgs Boson Coupling to the Top Quark in the Higgs to Diphoton Decay Channel |
Author(s) | Dickinson, Jennet Elizabeth |
Publication | Cham, Springer International Publishing, 2021. |
Description | XIV, 223 p. 146 illus., 130 illus. in color : online resource |
Abstract Note | During Run 2 of the Large Hadron Collider, the ATLAS experiment recorded proton-proton collision events at 13 TeV, the highest energy ever achieved in a collider. Analysis of this dataset has provided new opportunities for precision measurements of the Higgs boson, including its interaction with the top quark. The Higgs-top coupling can be directly probed through the production of a Higgs boson in association with a top-antitop quark pair (ttH). The Higgs to diphoton decay channel is among the most sensitive for ttH measurements due to the excellent diphoton mass resolution of the ATLAS detector and the clean signature of this decay. Event selection criteria were developed using novel Machine Learning techniques to target ttH events, yielding a precise measurement of the ttH cross section in the diphoton channel and a 6.3 $\sigma$ observation of the ttH process in combination with other decay channels, as well as stringent limits on CP violation in the Higgs-top coupling |
ISBN,Price | 9783030863685 |
Keyword(s) | 1. EBOOK
2. EBOOK - SPRINGER
3. Elementary particles (Physics)
4. Elementary Particles, Quantum Field Theory
5. MACHINE LEARNING
6. PARTICLE PHYSICS
7. PARTICLES (NUCLEAR PHYSICS)
8. QUANTUM FIELD THEORY
|
Item Type | eBook |
Multi-Media Links
Please Click here for eBook
Circulation Data
Accession# | |
Call# | Status | Issued To | Return Due On | Physical Location |
I11910 |
|
|
On Shelf |
|
|
|
|
29.
|
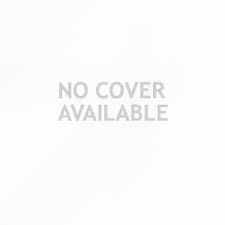 |
Title | Higgs Boson Decays into a Pair of Bottom Quarks : Observation with the ATLAS Detector and Machine Learning Applications |
Author(s) | Tosciri, Cecilia |
Publication | Cham, Springer International Publishing, 2021. |
Description | XIX, 159 p. 84 illus., 76 illus. in color : online resource |
Abstract Note | This thesis presents the analysis that led to the observation of the Standard Model (SM) Higgs boson decay into pairs of bottom quarks. The analysis, based on a multivariate strategy, exploits the production of a Higgs boson associated with a vector boson. The analysis was performed on a dataset corresponding to a luminosity of 79.8/fb collected by the ATLAS experiment during Run-2 at a centre-of-mass energy of 13 TeV. An excess of events over the expected background is observed in a combination with complementary Hbb searches. The analysis was extended to provide a finer interpretation of the signal measurement. The cross sections of the V H(H ??? bb) process have been measured in exclusive regions of phase space and used to search for deviations from the SM with an effective field theory approach. The results are discussed in this book. A novel technique for the fast simulation of the ATLAS forward calorimeter response is also presented. The new technique is based on similarity search, a branch of machine learning that enables quick and efficient searches for vectors similar to each other |
ISBN,Price | 9783030879389 |
Keyword(s) | 1. EBOOK
2. EBOOK - SPRINGER
3. Elementary particles (Physics)
4. Elementary Particles, Quantum Field Theory
5. MACHINE LEARNING
6. PARTICLE PHYSICS
7. PARTICLES (NUCLEAR PHYSICS)
8. QUANTUM FIELD THEORY
|
Item Type | eBook |
Multi-Media Links
Please Click here for eBook
Circulation Data
Accession# | |
Call# | Status | Issued To | Return Due On | Physical Location |
I11867 |
|
|
On Shelf |
|
|
|
|
30.
| 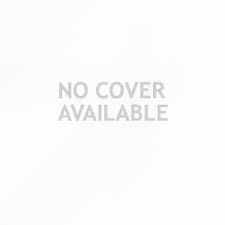 |
Title | Machine Learning with Quantum Computers |
Author(s) | Schuld, Maria;Petruccione, Francesco |
Publication | Cham, Springer International Publishing, 2021. |
Description | XIV, 312 p. 104 illus., 74 illus. in color : online resource |
Abstract Note | This book offers an introduction into quantum machine learning research, covering approaches that range from "near-term" to fault-tolerant quantum machine learning algorithms, and from theoretical to practical techniques that help us understand how quantum computers can learn from data. Among the topics discussed are parameterized quantum circuits, hybrid optimization, data encoding, quantum feature maps and kernel methods, quantum learning theory, as well as quantum neural networks. The book aims at an audience of computer scientists and physicists at the graduate level onwards. The second edition extends the material beyond supervised learning and puts a special focus on the developments in near-term quantum machine learning seen over the past few years |
ISBN,Price | 9783030830984 |
Keyword(s) | 1. EBOOK
2. EBOOK - SPRINGER
3. MACHINE LEARNING
4. MATHEMATICS
5. Mathematics and Computing
6. QUANTUM COMPUTERS
7. Quantum computing
|
Item Type | eBook |
Multi-Media Links
Please Click here for eBook
Circulation Data
Accession# | |
Call# | Status | Issued To | Return Due On | Physical Location |
I11858 |
|
|
On Shelf |
|
|
|
| |